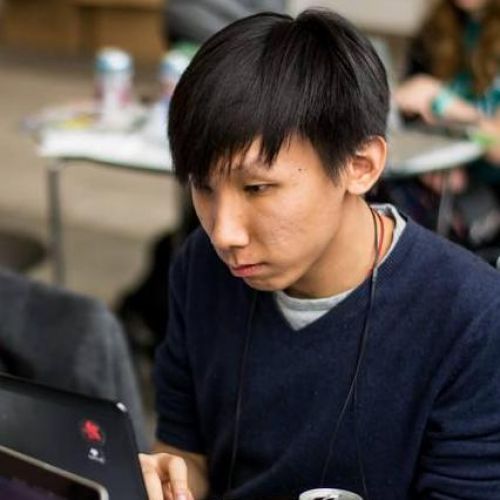
Sizhe Yuen is a final year PhD Student at the University of Southampton. His work focuses on the development of Evolutionary Algorithms using modern biological concepts from the Extended Evolutionary Synthesis. He has also worked on using machine learning to predict behavioural patterns in Dota 2 players. He graduated in 2019 from the University of St Andrews with an MSci (Hons) in Computer Science. His research is supported by the Southampton Marine and Maritime Institute.
Abstract:
Hundreds of Evolutionary Computation approaches have been reported. From an evolutionary perspective they focus on two fundamental mechanisms: cultural inheritance in Swarm Intelligence and genetic inheritance in Evolutionary Algorithms. Contemporary evolutionary biology looks beyond genetic inheritance, proposing a so-called "Extended Evolutionary Synthesis". Many concepts from the Extended Evolutionary Synthesis have been left out of Evolutionary Computation as interest has moved towards specific implementations of the same general mechanisms.
One such concept is epigenetic inheritance. Using a contemporary biological framework based on the Extended Evolutionary Synthesis, epigenetic inheritance is shown as a missing gap despite being a key building block in modern interpretations of how evolution occurs. Epigenetic mechanisms allow quick non- or partially-genetic adaptations to environmental changes. Dynamic multi-objective optimization problems represent similar circumstances to the natural world where fitness can be determined by multiple objectives (traits), and the environment is constantly changing.
We ask if the advantages that epigenetic inheritance provide in the natural world are replicated in dynamic multi-objective optimization problems. Specifically, an epigenetic blocking mechanism is applied to a state-of-the-art multi-objective genetic algorithm and its performance is compared on three sets of dynamic test functions. The mechanism shows improved performance on 12 of the 16 test problems, providing initial evidence that more algorithms should explore the wealth of epigenetic mechanisms seen in the natural world. These studies are extended to a practical problem, to demonstrate the real-world applicability of such an approach